Some context to the landscape
The fist pictogram has a list to the right that showcases what google currently suggests for me as automated fill out when I type a search query, for example when I start typing ‘How to’ or ‘Why do’ and it attempts to fill in the rest of the sentence. This is different for every person, but my intention with showing this is to start with what google already knows about me and is suggesting I should want to find, and then try to reverse engineer how they got there based on available data (not all of it is available) to get a general idea of how I’m being profiled. Unsurprisingly, all the things that are suggested at present have to do with topics currently going on in my life some of which are highlighted on Pictogram 1, and not all of which I searched for directly in google. Some of these are even things like my mother getting a new dog, mi intention to quit social media addiction, recent acquisition of electronics, etc.
The data sets I’m using are from both google chrome extension (which also harnesses your data even if you’re using a search engine that isn’t google) and the google maps data set.
My past study was related to the same subject, only it was using my youtube data so my intention is also to expand on how I’m spending my time on these platforms as well.
I realize the question is a bit ambitious since I’m only a beginner, and the people in this space are entire teams of sophisticated engineers, however, some interesting insights might surface along the way.
Audience
My audience could be divided into 2 categories: The more apt audience to criticize and give feedback to my work are engineering professionals or data savvy analysts that can better guide me through my learning curve as I am still learning the language of data and programing. They might find the question relevant or interesting as a challenge to try and solve themselves and find holes in this work.
On the other hand we have people like myself who just have general understanding of data, but who have an interest in disclosing the procedures Google has deployed for profiling us and what might actually be involved in that process.
Hypothesis/Assumptions
1.I would expect a long list of predetermined FAQ’s that contains keywords relating to my question, specially since as the words change
2.I expect seasonal questions, or time sensitive variations to be on top of the list. Ex: How cold is the weather tomorrow? would be on top.
3.Pattern recognition of my behavior: They probably know if I have repetitive behavior so they suggest it for me before I even search for it. Ex:Searching the same song over and over.
4.Actual number of views of news may vary, since sometimes I’m looking at them either on the phone or on facebook
5.They use my IP address to connect with credit cards purchases (Yes they store that too with Google Pay) and see if I purchased a new set of headphones, then they suggest how to connect them to my computer in the search bar.
6.Text analysis might be so advanced now that Google might even recognize the intent of making a question, and help clarify what you want.
7.Some of these suggestions in the search bar might be intended to provoke curiosity, to keep the user on longer than usual.
Google Maps Data
I’ve had a dramatic decrease in spatial searches from 2019 to 2020 according to data from google maps, which makes sense since I’ve traveled less, gone out less, eaten out less due to the COVID pandemic. Most searches in 2019 related to restaurants during the summer time, and also cultural activities. 2020 data is almost non-existent.
Google Chrome Data
Although It’s not possible to get a full picture of the google search bar suggestions from just the chrome extension data, and given that the topics I’ve searched are so varied, I classified everything into 2 different categories: Self improvement and Social media which is my biggest consumption ( I’m a bit embarrassed to admit this). I also used just the last month since I don’t yet have the skills to classify entire years worth of data.
I’m starting a master’s degree and lot of my searches have to do with email, tuition, student loans, registration, learning so I classified all that into self improvement.
From a personal standpoint, the amount of increase in social media consumption may be equal to the amount of time spent outside before the pandemic, and although this claim isn’t based on data, it’s probably been my response to the lack of social interaction, since I didn’t consume that much before.
Conclusions and next steps
It’s difficult to try and figure out how an algorithm that has many years in the making, that is proprietary and that uses a lot of data in different ways actually works for a small work like this one. I wasn’t able to find any data conclusive as to how they knew I wanted to delete my social media accounts, how they knew dogs might be a relevant subject in my life recently, etc. Since none of that input was typed into chrome.
Doing text analysis or word bubbles might be a next step, or having coding abilities and automating text analysis to figure out what was that secure would be my best bet.
My social media consumption has gone way up in the past year, and the mechanisms behind that addiction are still a mystery to me.
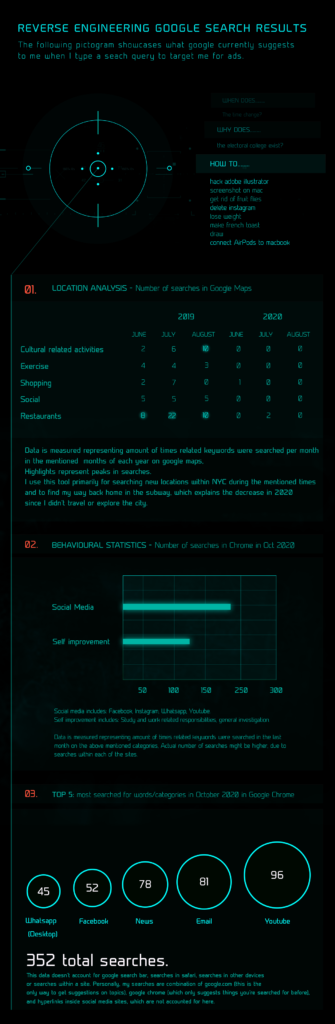